Abstract
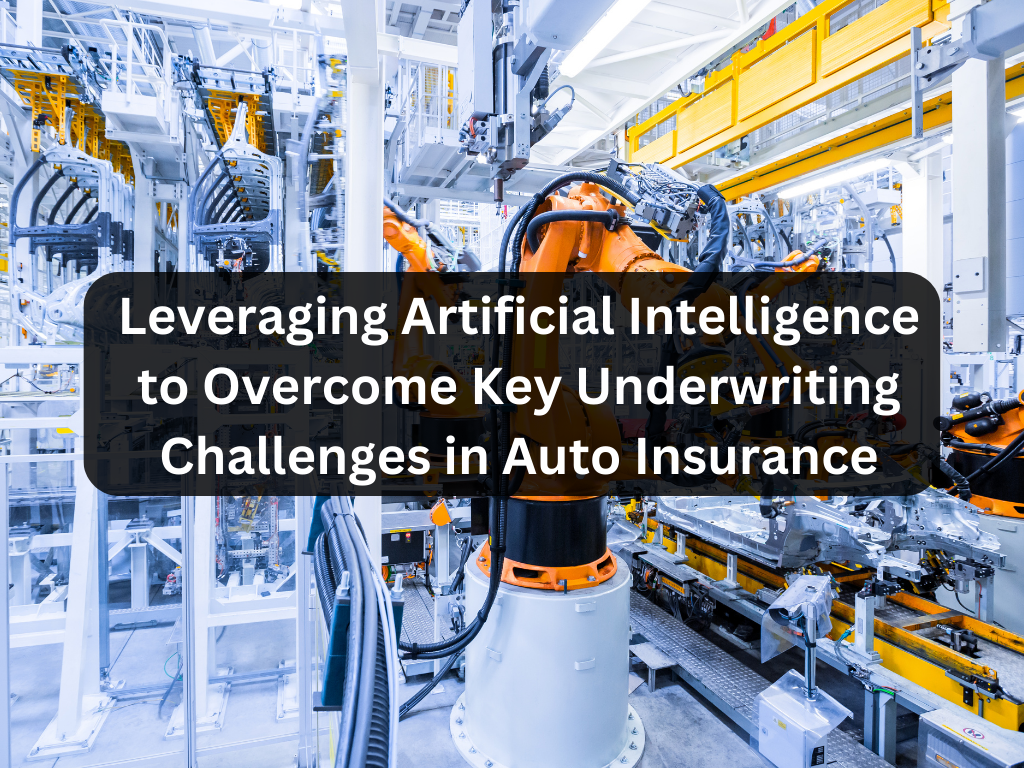
This whitepaper examines how Artificial Intelligence (AI) addresses critical challenges in auto insurance underwriting, including inaccurate risk assessment, inefficiencies in the underwriting process, staff turnover, outdated methodologies, and limited data in new markets. By implementing AI, insurers can enhance decision-making, streamline workflows, and open new growth avenues in underserved markets.
Key Challenges in Auto Insurance Underwriting
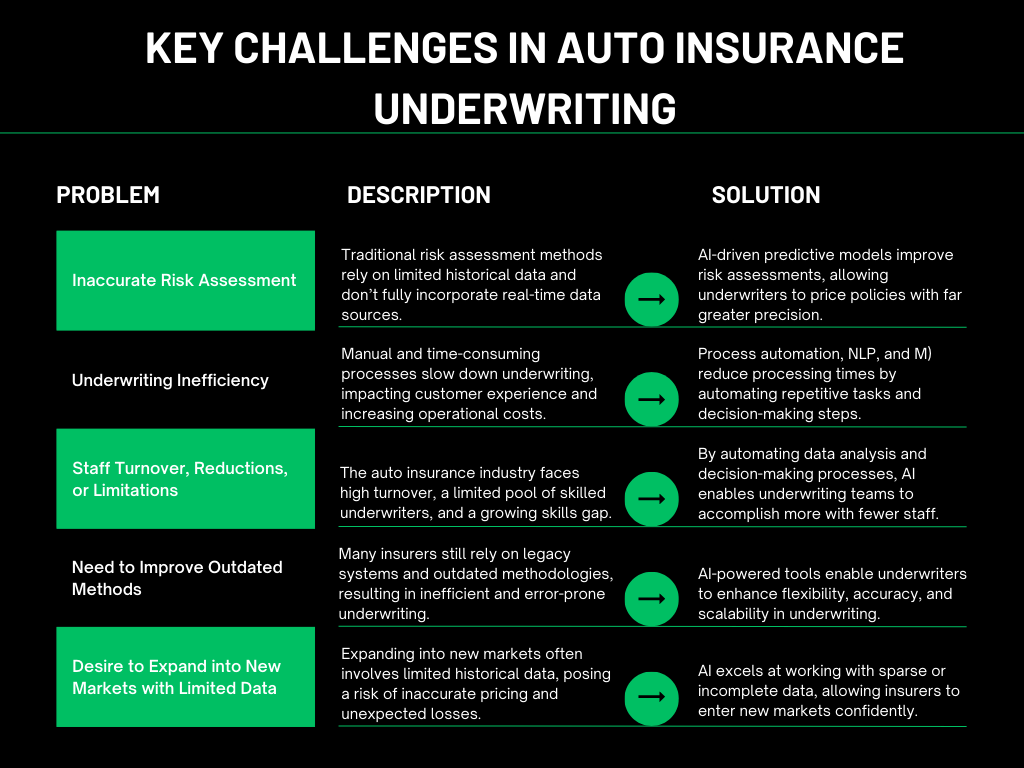
- Inaccurate Risk Assessment
- Problem: Traditional risk assessment methods rely on limited historical data and don’t fully incorporate real-time data sources, leading to inaccuracies and suboptimal premium pricing.
- AI Solution: AI-driven predictive models improve risk assessments by integrating data from telematics, IoT devices, and behavior analytics, allowing underwriters to price policies with far greater precision.
- Underwriting Inefficiency
- Problem: Manual and time-consuming processes slow down underwriting, impacting customer experience and increasing operational costs.
- AI Solution: Process automation, natural language processing (NLP), and machine learning (ML) reduce processing times by automating repetitive tasks and decision-making steps, leading to faster policy issuance and lower costs.
- Staff Turnover, Reductions, or Limitations
- Problem: The auto insurance industry faces high turnover, a limited pool of skilled underwriters, and a growing skills gap due to rapid technological change.
- AI Solution: By automating data analysis and decision-making processes, AI enables underwriting teams to accomplish more with fewer staff. Augmenting staff capabilities with AI also helps bridge skill gaps and reduces the dependency on specialized expertise.
- Need to Improve Outdated Methods
- Problem: Many insurers still rely on legacy systems and outdated methodologies that fail to capture the benefits of modern data analytics, resulting in inefficient and error-prone underwriting.
- AI Solution: AI-powered tools enable underwriters to transition from static, rule-based models to adaptive, data-driven models, enhancing flexibility, accuracy, and scalability in underwriting.
- Desire to Expand into New Markets with Limited Data
- Problem: Expanding into new geographic or demographic markets often involves limited historical data, posing a risk of inaccurate pricing and unexpected losses.
- AI Solution: AI excels at working with sparse or incomplete data by using transfer learning and synthetic data generation, allowing insurers to enter new markets confidently, even with limited historical information.
AI-Driven Solutions for Addressing Underwriting Challenges
- Enhanced Data Processing and Integration
- Use of Machine Learning (ML) Models to synthesize data from telematics, customer interactions, and third-party sources, enabling more accurate risk profiles.
- Natural Language Processing (NLP) to analyze unstructured data sources, such as customer notes and social media, for additional insights into driver behavior.
- Automating Repetitive Underwriting Tasks
- Robotic Process Automation (RPA) and AI workflows for document analysis, data entry, and verification to streamline underwriting and reduce processing time.
- AI-enabled fraud detection systems to identify inconsistencies in applications, helping reduce underwriting workload.
- AI Augmentation of Underwriting Talent
- Implementing AI-based tools to support less experienced underwriters, allowing them to make informed decisions with the aid of machine recommendations.
- Leveraging decision-support systems to increase underwriters’ capacity, helping mitigate the impact of workforce limitations or turnover.
- Transitioning from Legacy Systems to AI-Driven Underwriting
- AI models improve legacy processes by analyzing historical and real-time data, and using predictive analytics to refine pricing models.
- Machine learning algorithms that continually adapt based on emerging data, helping companies transition from static methods to dynamic, data-driven underwriting practices.
- Expanding into New Markets Using AI
- AI-based transfer learning allows insurers to apply knowledge from one market to a new market with limited data.
- Synthetic Data Generation to create representative datasets where real data is lacking, helping reduce the uncertainty of underwriting in untested markets.
Implementing AI for Underwriting in Auto Insurance
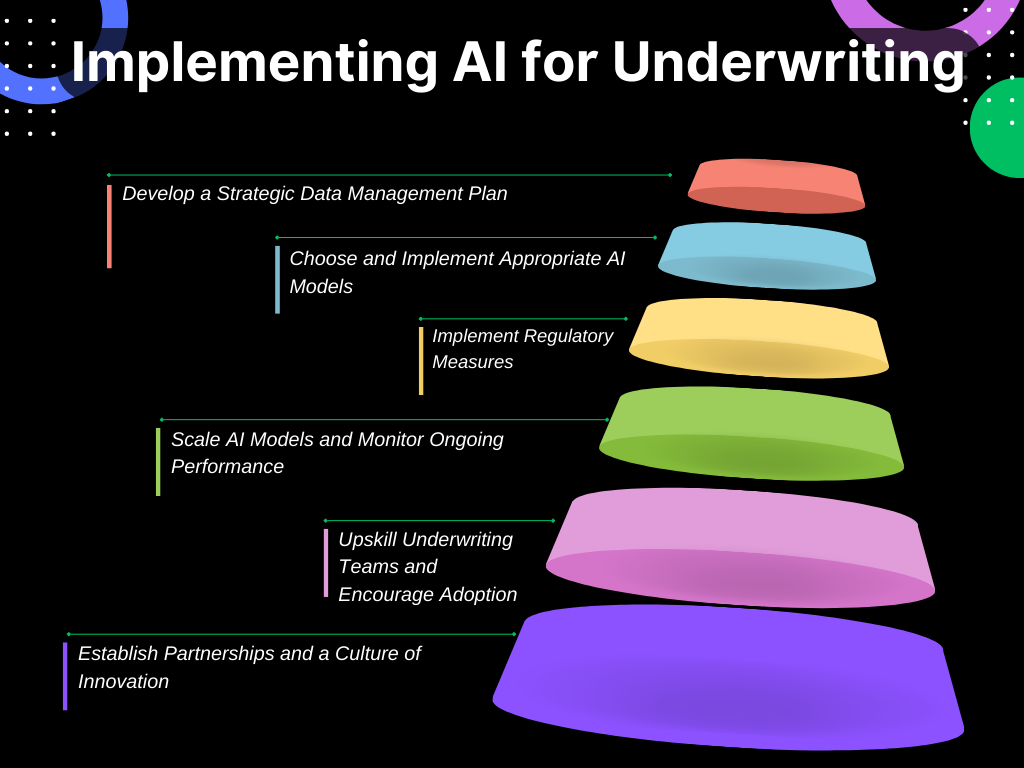
Step 1: Develop a Strategic Data Management Plan
- Identify Key Data Sources: List and prioritize relevant data sources, such as telematics, IoT, customer history, and external risk indicators.
- Set Up a Data Pipeline: Use cloud infrastructure to gather, integrate, and store these data sources. Ensure you select a cloud provider with strong security and compliance standards.
- Establish Data Quality and Consistency Checks: Create standards for data accuracy, consistency, and completeness to ensure high-quality inputs for AI models.
Step 2: Choose and Implement Appropriate AI Models
- Define Underwriting Needs and Objectives: Identify specific areas where AI will add value, such as risk assessment, premium calculation, or fraud detection.
- Select Suitable Machine Learning Algorithms: Choose algorithms aligned with your objectives. For instance:
- Use regression models for risk scoring.
- Apply classification models for binary decisions (e.g., approval vs. rejection).
- Set Model Performance Metrics: Define key metrics like accuracy, precision, recall, and F1 score to evaluate the model’s effectiveness.
- Pilot the AI Model on Historical Data: Run initial tests on past data to assess model performance, making adjustments as needed.
Step 3: Implement Ethical and Regulatory Compliance Measures
- Develop a Compliance Checklist: Identify applicable regulations (e.g., GDPR for data privacy, local insurance regulations) and ensure each one is met.
- Incorporate Explainable AI (XAI) Components: Choose models or techniques that provide interpretability, so underwriters and clients can understand AI-driven decisions.
- Conduct a Bias Audit: Test models for potential biases (e.g., gender, age) to ensure fairness and ethical standards.
Step 4: Scale AI Models and Monitor Ongoing Performance
- Deploy Models in a Controlled Environment: Start by implementing AI in specific underwriting cases, assessing the impact before scaling further.
- Set Up Continuous Monitoring and Feedback Loops: Track model performance in real-time, using feedback from underwriting teams to make adjustments.
- Regularly Update Models with New Data: Use fresh data to retrain models, ensuring they remain accurate and relevant over time.
Step 5: Upskill Underwriting Teams and Encourage Adoption
- Create Training Modules on AI Basics and Use Cases: Develop training resources to explain AI, focusing on how underwriters can use insights effectively.
- Offer Practical Workshops: Conduct hands-on sessions where underwriters can practice using the AI tools with real case studies.
- Establish Clear Support Channels: Set up a dedicated team or partner with a technology provider to offer ongoing support and address any technical issues quickly.
Step 6: Establish Partnerships and a Culture of Innovation
- Identify AI and Tech Partners: Collaborate with AI vendors or cloud providers with proven expertise in insurance.
- Plan Regular Innovation Review Meetings: Meet with partners periodically to discuss new technologies or improvements that could enhance the underwriting process.
- Encourage Knowledge Sharing: Host workshops, presentations, or informal meet-ups to keep underwriting teams updated on the latest AI developments and ideas.
Future Trends in AI for Auto Insurance Underwriting
- Behavioral Modeling for Personalized Policies: Real-time personalization based on driver behavior and data analytics.
- Blockchain for Secure Data Sharing: Ensuring a transparent and secure data flow across underwriting workflows.
- AI-Enhanced Risk Pooling: Using AI to pool risks across diverse markets and demographics dynamically.
Conclusion
Artificial Intelligence offers tremendous potential to address the five core challenges in auto insurance underwriting. By tackling issues such as inaccurate risk assessments, underwriting inefficiencies, workforce limitations, outdated methods, and data scarcity in new markets, AI can help insurers transition from reactive processes to proactive, data-driven approaches. This transformation not only reduces errors and speeds up decision-making but also enables insurers to assess risk with far greater precision and adapt more quickly to emerging market needs.
For auto insurers to stay competitive and meet rising customer expectations, strategically adopting AI-driven solutions is no longer optional—it’s essential. Leveraging AI in underwriting can enhance operational efficiency, improve customer satisfaction through faster processing times, and ensure pricing accuracy that meets regulatory standards. Insurers ready to invest in AI will be well-positioned to capitalize on new market opportunities, scale effectively, and deliver tailored customer experiences that set them apart in a rapidly evolving industry. Now is the time for insurers to embrace AI as the strategic enabler that will drive future growth and innovation in auto insurance underwriting.