Early Foundations: The Birth of AI (1950s-1960s)
The fascination with intelligent machines isn’t new. Stories of automatons appear in ancient myths, but it wasn’t until the mid-20th century that AI began to take scientific shape. A defining moment came in 1950 when British mathematician Alan Turing published his groundbreaking paper, “Computing Machinery and Intelligence.” Turing introduced the famous Turing Test, which aimed to determine whether a machine could exhibit behavior indistinguishable from that of a human. This concept of machines “thinking” ignited the imagination of scientists, setting the stage for AI’s future development.
In 1956, AI was officially born when John McCarthy coined the term at the Dartmouth Conference. Early AI pioneers like Marvin Minsky and Herbert Simon envisioned machines that could replicate human reasoning. However, early AI systems were rudimentary, relying on symbolic AI—essentially, rule-based systems that processed information in a rigid, predefined way. These early efforts, while foundational, were limited by the technology of the time.
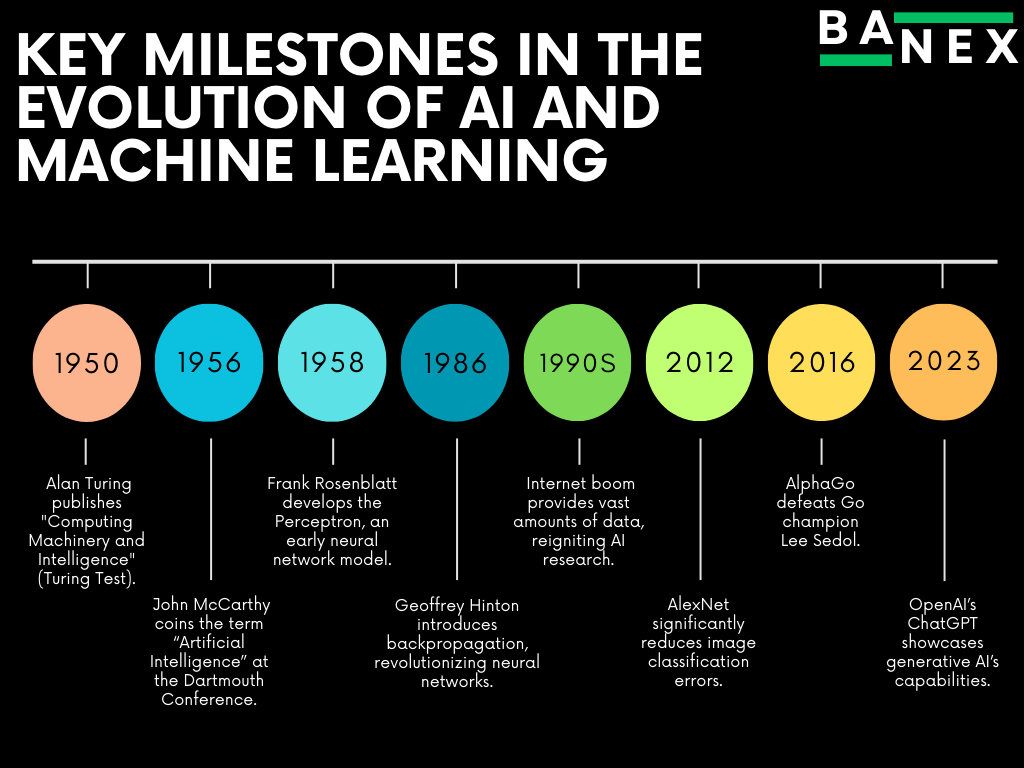
Machine Learning Emerges (1980s-1990s)
Alongside AI, the concept of machine learning—allowing machines to learn from data rather than strict programming—was slowly gaining traction. In 1958, Frank Rosenblatt introduced the Perceptron, an early neural network that could “learn” through feedback. However, neural networks didn’t gain significant momentum until decades later due to computational limitations.
By the 1980s, thanks to increased computational power and new algorithms, interest in neural networks was reignited. A breakthrough came with the development of backpropagation by Geoffrey Hinton and his team, which allowed multi-layer neural networks to “learn” by adjusting internal parameters in response to errors. This ability to improve through feedback set the foundation for more complex learning tasks and marked the true beginning of modern machine learning.
AI Winter: Setbacks and Slowdowns (1970s-1990s)
Despite early progress, the journey of AI and ML wasn’t without challenges. Throughout the 1970s to the 1990s, the AI community faced periods of disillusionment known as “AI Winters.” During these periods, funding dried up, and interest waned due to over-promised capabilities and under-delivered results. Early AI systems struggled to handle real-world complexity because they relied heavily on rule-based logic and lacked access to the large datasets and computational power we have today.
One significant factor in AI’s resurgence was the internet boom in the 1990s, which provided a massive influx of data. As more information became available, machine learning algorithms had the “fuel” they needed to evolve. The development of more sophisticated computational tools and data sources helped revive AI research, particularly ML.
The Era of Big Data and Deep Learning (2000s-present)
The 2000s marked a new era for AI and ML. The rise of the internet, social media, and cloud computing generated vast amounts of data, creating the perfect environment for these technologies to thrive. AI shifted from being rule-based to data-driven, allowing machine learning algorithms to improve with every new data point they processed.
Deep learning, a subset of machine learning, emerged as the dominant force. These models, based on neural networks with multiple layers, allowed machines to excel at complex tasks like image recognition, language processing, and autonomous driving. Convolutional Neural Networks (CNNs) and Recurrent Neural Networks (RNNs) gave AI systems the ability to “see” and “understand” data in ways that mimic human cognitive functions.
Key breakthroughs include:
- 2012: AlexNet, a deep learning model, won the ImageNet competition by dramatically improving image classification accuracy, and reducing error rates by over 25%.
- 2016: Google DeepMind’s AlphaGo defeated Go champion Lee Sedol, demonstrating AI’s capability to handle strategic games previously thought too complex for machines.
- 2023: OpenAI’s ChatGPT showcased AI’s potential to engage in natural, human-like conversation, opening new possibilities in human-AI interaction.
AI and ML Today: Transforming Industries
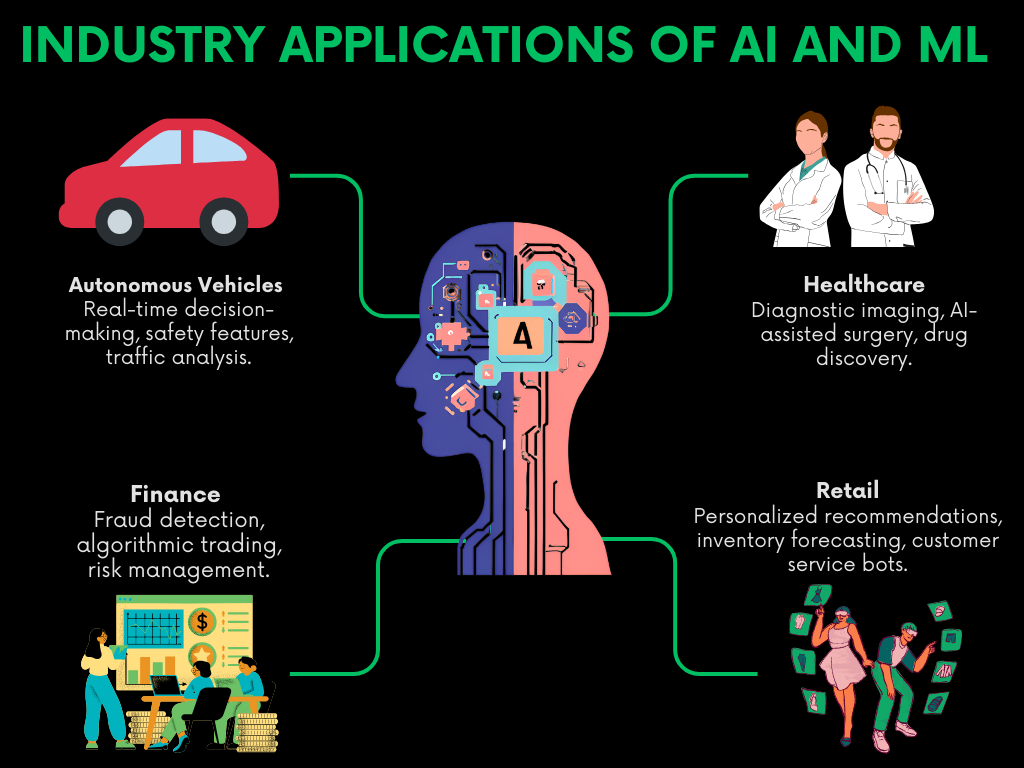
Today, AI and ML are integrated into nearly every industry, driving unprecedented levels of innovation, efficiency, and automation.
- Healthcare: AI is revolutionizing the field, particularly in diagnostics. According to Accenture, AI applications could save the U.S. healthcare economy up to $150 billion annually by 2026. For example, Google’s DeepMind developed an AI system that can detect over 50 different eye diseases with 94% accuracy, outperforming human specialists in some cases. AI also plays a key role in drug discovery, reducing the time it takes to develop new treatments.
- Finance: Machine learning models have become indispensable for detecting fraudulent transactions and predicting market trends. JPMorgan Chase uses its AI platform, COiN, to analyze legal contracts, saving 360,000 hours of manpower each year. AI is also behind advanced algorithmic trading strategies, helping firms optimize investment decisions in real-time.
- Retail: In e-commerce, AI personalizes shopping experiences at scale. Amazon attributes 35% of its revenue to its recommendation algorithms, which learn from user behavior to suggest products. AI also optimizes supply chains, forecasting demand with remarkable precision.
- Autonomous Vehicles: Self-driving car technology, powered by AI, is becoming increasingly sophisticated. Companies like Waymo and Tesla have logged millions of autonomous miles, with AI processing data from sensors to make split-second decisions. Studies predict that AI-powered vehicles could reduce traffic accidents by up to 90%, potentially saving thousands of lives and billions in healthcare costs annually.
Ethical Considerations and Challenges
Despite the benefits, AI’s rapid evolution also raises critical ethical questions. Concerns around algorithmic bias, data privacy, and the displacement of jobs are central to the ongoing debate.
- Algorithmic Bias: One major issue is the bias that can result from skewed training data. For instance, Amazon had to scrap an AI recruiting tool after it was discovered that the system discriminated against female candidates. As AI becomes more integrated into high-stakes decisions—like hiring or law enforcement—the consequences of biased algorithms grow more serious.
- Job Displacement: AI is expected to automate many jobs in sectors ranging from manufacturing to customer service. The World Economic Forum predicts that while AI could displace 85 million jobs by 2025, it could also create 97 million new roles, primarily in AI development, data science, and tech infrastructure. However, the transition poses challenges in reskilling the workforce.
Addressing these ethical challenges will be critical as AI continues to evolve. Researchers and policymakers must work together to create transparent, accountable systems that benefit society while minimizing harm.
Conclusion
The history of AI and ML is one of ambition, innovation, and perseverance. From the early theoretical work of Alan Turing to the deep learning breakthroughs that shape today’s world, AI has evolved from a niche field into a major driver of global innovation. Today, AI and ML are not just research topics—they are shaping the future of entire industries.
Looking ahead, AI’s potential is vast. It’s poised to address some of the world’s most pressing problems, from climate change to healthcare challenges. However, the journey forward must be tempered with caution. Ethical considerations, transparency, and responsible AI development will be essential to ensuring that these technologies serve humanity’s best interests.
As AI continues to evolve, it will push the boundaries of what machines—and humanity—can achieve, shaping the future in ways we are only beginning to understand.