Abstract
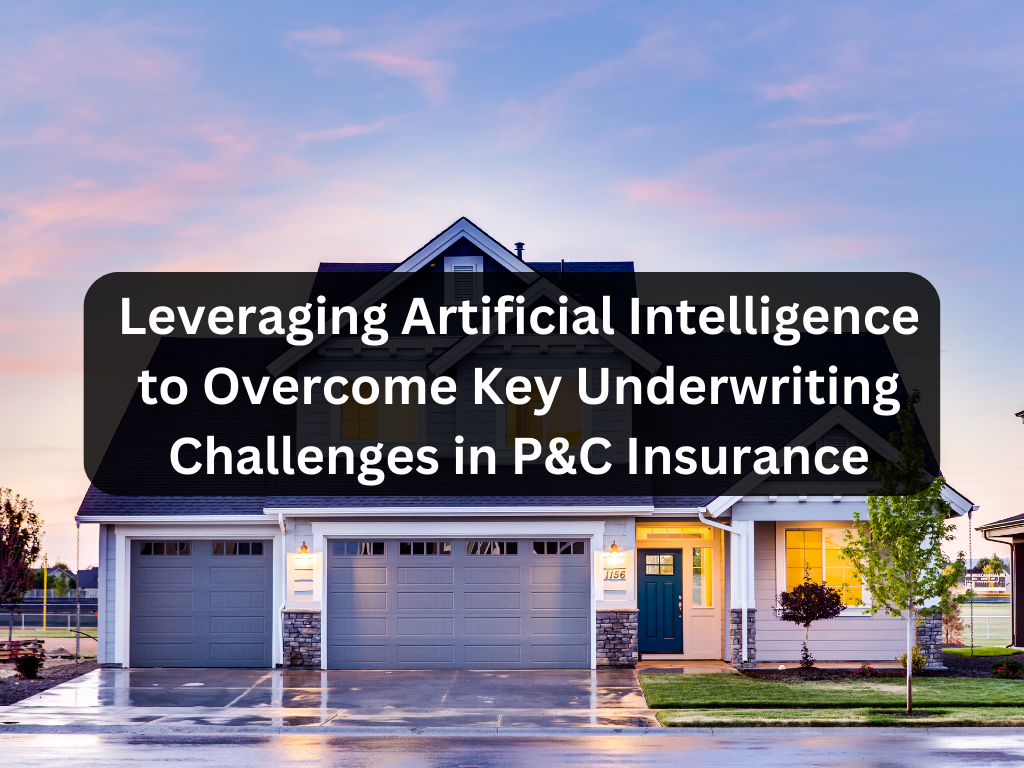
In today’s rapidly evolving insurance landscape, Property & Casualty (P&C) underwriting faces numerous challenges. From managing vast amounts of data to accurately assessing complex risks, the traditional underwriting process often falls short of modern demands. This paper explores how AI-driven approaches can address these issues by improving predictive accuracy, streamlining processes, enhancing fraud detection, and ensuring regulatory compliance. By examining the specific underwriting pain points and the transformative potential of AI, we provide actionable insights for insurers aiming to future-proof their underwriting operations.
Introduction
The State of P&C Underwriting
The Property & Casualty insurance market is crucial to providing financial protection against losses, with a global market size of approximately $1.5 trillion as of 2022. This sector continues to grow, with annual premiums expected to increase by 3.5% annually due to expanding risks, particularly climate-related ones (Allied Market Research, 2023). However, the accuracy and efficiency of underwriting are under increasing pressure as risks become more complex and data sources more abundant. Underwriters must navigate a constantly shifting environment, where mispricing or failure to identify risk could result in significant losses, a concern highlighted by the fact that approximately 40% of insurers report suboptimal risk assessment due to data challenges (McKinsey & Company, 2022).
Challenges in Traditional Underwriting
Traditional underwriting often relies on historical data, manual processes, and industry-standard risk models, which struggle to keep pace with the complexity of emerging risks. With insurers managing more than 2.5 quintillion bytes of data daily (IDC, 2023), traditional processes fall short in leveraging unstructured data sources such as satellite imagery, social media, and IoT. Additionally, manual processing leads to costly delays, with approximately 25% of claims experiencing significant lag time due to manual review processes (Deloitte, 2022). AI promises to revolutionize underwriting by streamlining processes, enhancing risk profiling, and reducing both costs and errors.
Key Underwriting Challenges in P&C Insurance
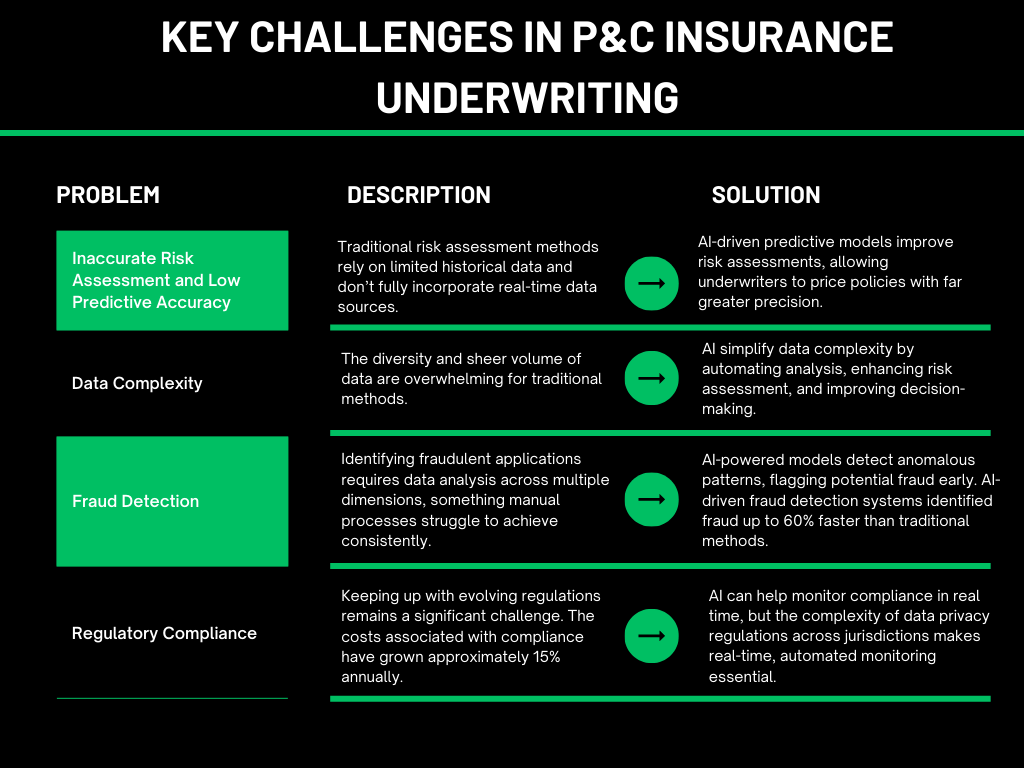
Risk Assessment and Predictive Accuracy
Risk assessment remains challenging, particularly given the unpredictability of catastrophic events, which have increased 44% over the past decade due to climate change (Swiss Re Institute, 2023). Traditional models often lack the granularity needed to predict specific risks accurately, resulting in coverage gaps. For example, around 35% of P&C insurers report challenges in accurately modeling natural disasters and property damage risks (Munich Re, 2022).
Data Complexity
The diversity and sheer volume of data are overwhelming for traditional methods. Data from sensors, historical loss records, and even customer behavior contribute to a complex environment where relevant information is often underutilized. An estimated 90% of data generated by P&C insurers is unstructured, such as customer emails or accident reports, which conventional systems struggle to process (IBM, 2023).
Fraud Detection
Fraudulent claims cost the P&C industry nearly $80 billion annually in the United States alone (FBI, 2022). Identifying fraudulent applications requires data analysis across multiple dimensions, something manual processes struggle to achieve consistently. Studies show that insurers using AI-powered fraud detection reduced losses by up to 20% within two years (Accenture, 2023).
Regulatory Compliance
Keeping up with evolving regulations remains a significant challenge. The costs associated with compliance have grown approximately 15% annually as regulatory scrutiny increases (PwC, 2023). AI can help monitor compliance in real-time, but the complexity of data privacy regulations across jurisdictions makes real-time, automated monitoring essential.
The Role of AI in Enhancing Underwriting
Data Enrichment
AI’s ability to process both structured and unstructured data allows insurers to incorporate previously untapped sources like IoT data, social media, and public records, enhancing predictive accuracy. For example, predictive AI models incorporating geospatial and historical weather data were found to increase underwriting accuracy by 30% (McKinsey & Company, 2023).
Predictive Analytics
AI algorithms enable granular risk assessment by analyzing data trends, which allows for more accurate predictions. In one study, AI-based predictive analytics reduced claims frequency by 18% through better risk selection and pricing (KPMG, 2023).
Natural Language Processing (NLP)
NLP is transforming underwriting by extracting valuable insights from unstructured data like accident reports and customer communications. For example, NLP-enabled systems processed 85% of claim documentation without human intervention, cutting processing time by nearly 60% (Forrester, 2023).
Machine Learning for Risk Scoring
Machine learning (ML) models use complex risk variables to create accurate risk scores that enable faster, data-driven underwriting. Studies show that ML-powered risk scoring improved policy renewal rates by 20% by offering customized premiums based on risk profiles (EY, 2023).
Automation & RPA
Robotic Process Automation (RPA) can streamline repetitive tasks, increasing the underwriting process speed by 40% (Deloitte, 2023). By freeing underwriters from administrative work, RPA allows them to focus on higher-value tasks, reducing underwriting cycle times by 30% on average.
AI-Driven Solutions for Key Underwriting Challenges
Risk Assessment Enhancement
AI algorithms analyze historical data and real-time information to create more precise risk profiles, especially valuable in high-risk areas such as natural disaster coverage. For instance, AI models used in risk assessment for hurricanes and wildfires improved predictive accuracy by nearly 40%, significantly reducing unexpected claims (Swiss Re Institute, 2023).
Real-Time Decision Making
AI can provide real-time insights into emerging risks, allowing insurers to make on-the-spot policy adjustments. This approach has been shown to reduce time-to-decision by 50% and decrease loss ratios by up to 15% (Capgemini, 2023).
Fraud Detection
AI-powered models detect anomalous patterns, flagging potential fraud early. In pilot studies, AI-driven fraud detection systems identified fraud up to 60% faster than traditional methods, preventing losses that would have otherwise impacted profitability (FBI, 2023).
Regulatory Compliance and Governance
By automating compliance monitoring, AI ensures that insurers stay up-to-date with regulatory changes, reducing compliance costs by up to 25% annually (PwC, 2023). Real-time data monitoring also assists in audits, ensuring transparency in underwriting decisions.
AI Implementation Roadmap for P&C Insurers
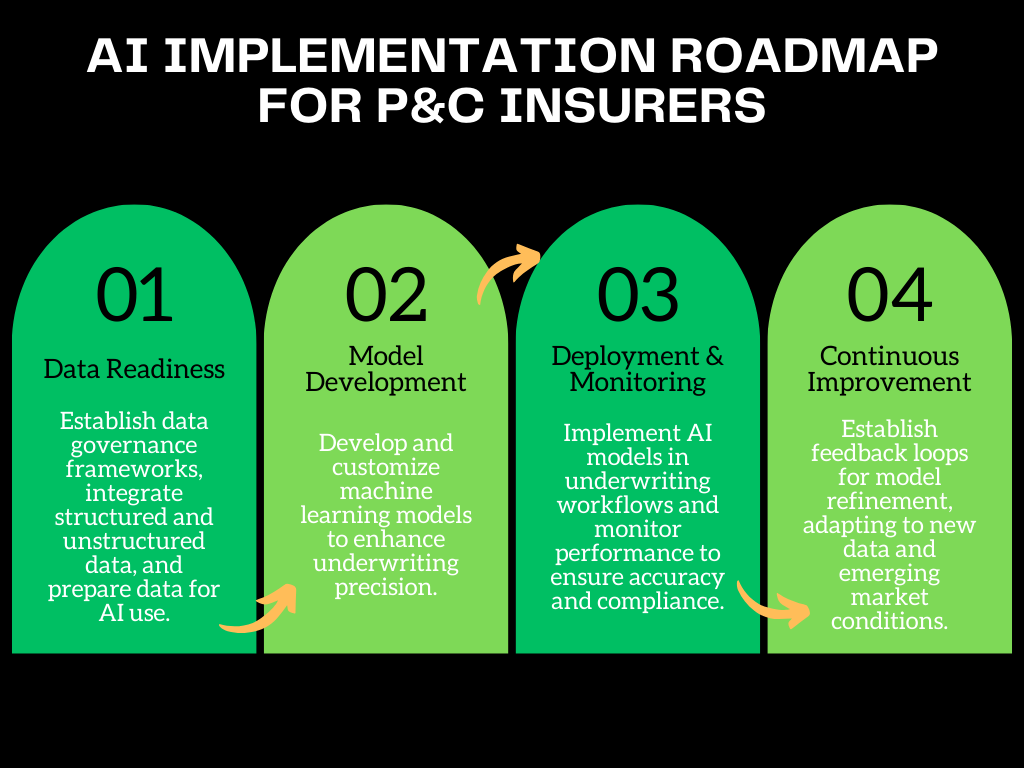
Phase 1: Data Readiness: Establish data governance frameworks, integrate structured and unstructured data, and prepare data for AI use.
Phase 2: Model Development: Develop and customize machine learning models to enhance underwriting precision.
Phase 3: Deployment & Monitoring: Implement AI models in underwriting workflows and monitor performance to ensure accuracy and compliance.
Phase 4: Continuous Improvement: Establish feedback loops for model refinement, adapting to new data and emerging market conditions.
Challenges and Risks of Implementing AI in Underwriting
- Data Privacy and Security: Ensuring data protection while complying with regional data privacy laws is critical as AI collects sensitive information.
- Model Transparency: Addressing the “black-box” challenge to ensure underwriters understand and trust AI-generated insights.
- Integration with Existing Systems: Challenges of integrating AI with legacy systems while training underwriters on the new processes.
- Talent & Skills Gap: The need for AI expertise in the insurance sector to build, deploy, and maintain these models.
Future Outlook for AI in P&C Underwriting
- IoT and Connected Devices: With the IoT market for insurance projected to grow to $42 billion by 2028 (Grand View Research, 2023), connected devices will continue to enrich data for risk assessment.
- Personalized Underwriting: AI enables insurers to tailor policies for individual risk profiles, driving customer satisfaction and profitability.
- Collaborative AI Systems: Augmented intelligence will allow underwriters to work alongside AI, increasing efficiency and enhancing customer experience.
Conclusion
AI is rapidly becoming essential to overcoming underwriting challenges in P&C insurance. By addressing inefficiencies, enhancing risk assessment, and ensuring compliance, AI not only drives operational efficiency but also empowers underwriters to meet the evolving demands of a data-rich, dynamic environment. Insurers investing in AI stand to gain a substantial competitive advantage, as this technology redefines the underwriting landscape.