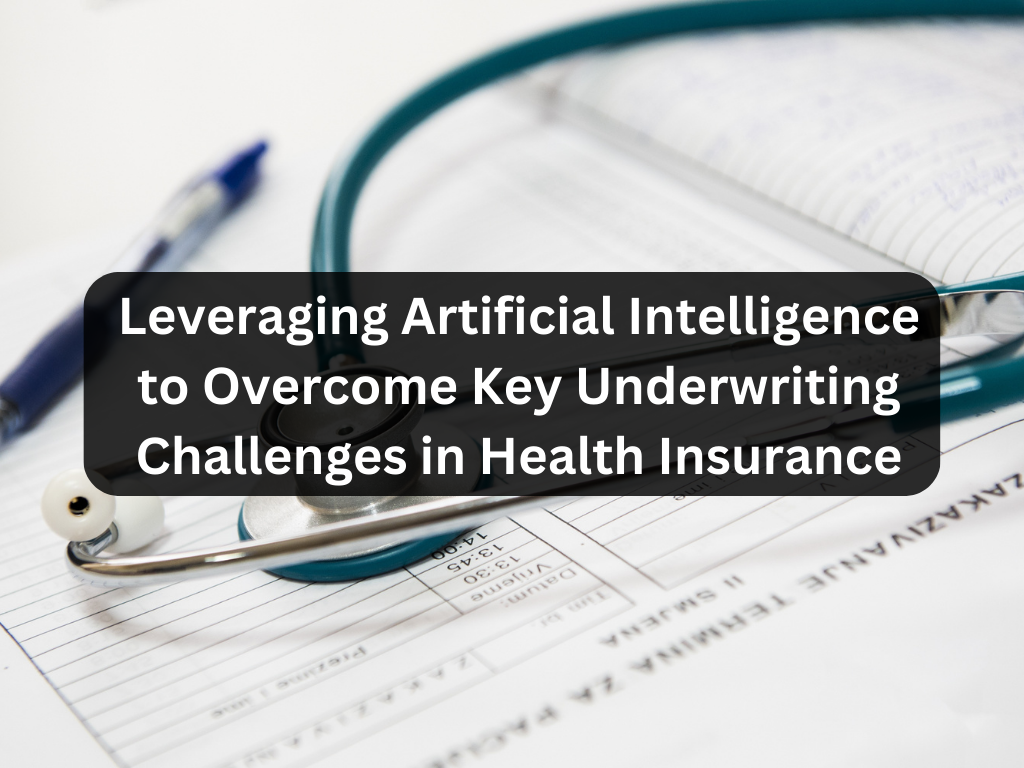
The healthcare insurance industry faces rising demand for cost-effective, precise underwriting solutions. According to McKinsey, insurers who adopt AI in underwriting have achieved processing times up to 70% faster, reducing costs by as much as 30%. Traditional methods, reliant on manual processes and subjective decision-making, struggle to meet data complexity and efficiency needs. This paper explores how AI transforms underwriting in healthcare insurance, enabling enhanced risk assessment, operational efficiency, and customer satisfaction.
Introduction
Underwriting in healthcare insurance requires in-depth data analysis to balance risk and affordability. Traditional underwriting processes are not only time-consuming but also error-prone, as they involve large amounts of unstructured health data. AI offers a significant edge: the global AI in insurance market, valued at $1.4 billion in 2020, is expected to grow at a CAGR of 24% through 2028. With advanced AI, insurers can automate and streamline underwriting, significantly improving risk assessment and operational efficiency.
Challenges in Healthcare Insurance Underwriting
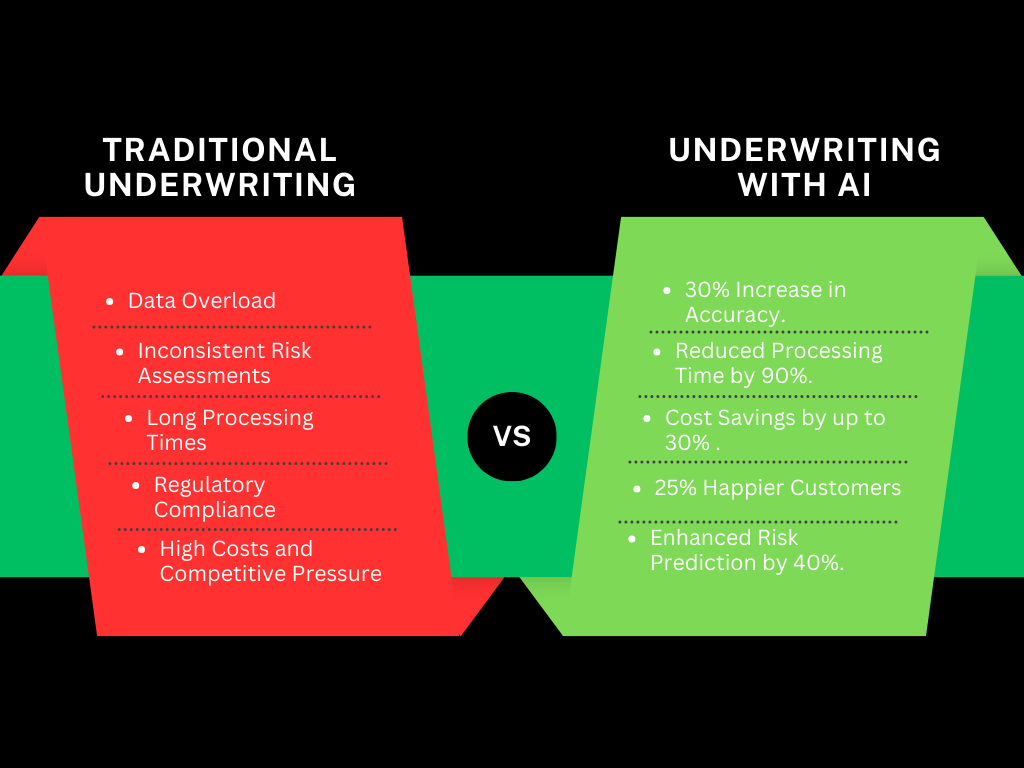
Data Overload
Healthcare data is growing rapidly, with global healthcare data projected to reach 2,314 exabytes by 2025, as stated by IDC in its Global Datasphere report. Underwriters face significant challenges in processing these volumes accurately and efficiently.
Inconsistent Risk Assessments
Manual underwriting leads to inconsistencies in risk outcomes, with a typical variance of 15-20%, resulting in pricing and coverage inaccuracies, according to Deloitte’s survey on insurance underwriting.
Long Processing Times
According to a 2021 report by Accenture, traditional underwriting processes can take 4-6 weeks per application. Studies show that automated AI underwriting can reduce this to a few days, or even hours, improving response times and customer satisfaction.
Regulatory Compliance
Stringent regulations such as HIPAA and GDPR create complexities in handling sensitive healthcare data. AI can help improve compliance with automated data privacy tools, reducing the risk of costly regulatory fines, which have amounted to over $1 billion in the U.S. alone since the introduction of HIPAA. (HHS.gov)
High Costs and Competitive Pressure
According to Deloitte, operational costs for insurers who rely on traditional underwriting have increased by nearly 10% annually. AI can cut these costs by 20-30%, keeping insurers competitive, as reported by PwC in their “Top Issues in Insurance” report.
Role of AI in Healthcare Insurance Underwriting
AI can revolutionize healthcare insurance underwriting by automating data processing, analyzing risk factors accurately, and enabling personalized risk assessments. The following sections detail how AI addresses each of the above challenges.
- Enhanced Data Processing and Analysis
AI-driven tools can process large volumes of unstructured data from diverse sources, such as electronic health records (EHRs), medical claims, and lifestyle data. Machine learning (ML) algorithms extract meaningful insights, enabling underwriters to make data-informed decisions efficiently.
- Natural Language Processing (NLP): NLP techniques analyze unstructured data, like physician notes or lab reports, providing underwriters with valuable insights that are otherwise challenging to extract. Studies show NLP can extract 80-90% of insights from unstructured data, such as medical notes, allowing more comprehensive risk assessments.
- Data Mining: AI-based data mining identifies patterns within historical data, supporting risk prediction and revealing underlying health trends.
- Predictive Analytics for Risk Scoring
Predictive modeling in AI enables underwriters to assess future health risks based on historical data. By evaluating an applicant’s likelihood of developing specific health conditions, AI tools provide personalized risk scores that contribute to accurate premium calculation.
- Chronic Disease Prediction: AI models identify applicants at risk of chronic illnesses, such as diabetes or cardiovascular diseases, allowing insurers to anticipate potential healthcare costs. AI models have achieved up to 85% accuracy in predicting chronic conditions such as diabetes, as demonstrated in IBM Watson Health’s pilot studies.
- Lifestyle Risk Assessment: AI-based risk models improve inaccuracy by approximately 20% by incorporating lifestyle data (Capgemini, 2020).
- Automation of Routine Underwriting Processes
AI can streamline the underwriting process by automating repetitive tasks, reducing human intervention, and expediting application approvals. Robotic Process Automation (RPA) integrated with AI allows insurers to handle data collection, verification, and preliminary risk assessments without manual input.
- Automated Document Processing: AI-driven OCR (Optical Character Recognition) converts paper-based health records into digital formats, enabling seamless integration into underwriting systems. OCR digitizes and organizes records with 95% accuracy, reducing data entry time, as shown in recent case studies by KPMG.
- Decision Support Systems: AI-powered decision support tools aid underwriters by recommending risk categories or premium ranges based on AI-driven risk assessments.
- Compliance and Data Privacy Management
AI tools are designed with privacy-preserving mechanisms to ensure regulatory compliance. Techniques like federated learning allow insurers to utilize AI for risk assessment while protecting sensitive health data.
- Data Anonymization: AI solutions anonymize sensitive data, allowing analysis without revealing personally identifiable information.
- Federated Learning: This technique enables insurers to train AI models using decentralized data, complying with privacy regulations by keeping data localized.
Benefits of AI-Powered Underwriting in Healthcare Insurance
Increased Accuracy
AI-driven risk assessments reduce inconsistencies, providing underwriters with consistent and accurate risk profiles. Due to consistent data analysis, AI-driven underwriting accuracy is up to 30% higher than traditional approaches, according to Forrester’s AI in Insurance study.
Reduced Processing Time
Automation and predictive analytics accelerate the underwriting process, enhancing customer experience by reducing wait times. According to Accenture, automated processes have reduced underwriting processing times from 4-6 weeks to 1-3 days on average.
Cost Savings
By automating routine tasks and improving decision accuracy, AI helps insurers lower operational costs and maintain competitive pricing. AI adoption in underwriting has led to cost reductions of up to 20-30%, as shown in a PwC report on insurance efficiencies.
Improved Customer Experience
Faster underwriting processes and tailored policies enhance customer satisfaction, making it easier for customers to obtain timely insurance coverage. As per Deloitte, faster application turnaround time and personalized pricing increased customer satisfaction by approximately 25%.
Enhanced Risk Prediction
Predictive analytics allow insurers to anticipate health risks more accurately, leading to better-informed pricing strategies and improved risk management. Predictive models improve risk prediction accuracy by 40%, as insurers can better align pricing with applicants (McKinsey, 2020).
Implementation Strategies for AI in Healthcare Underwriting
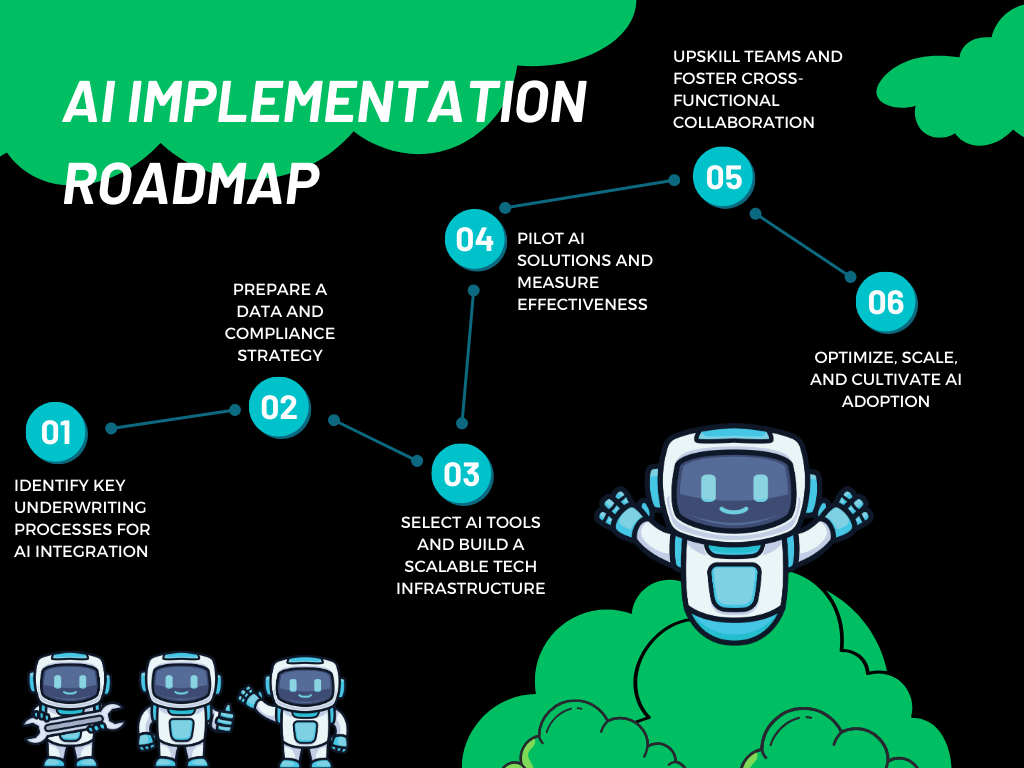
Step 1: Identify Key Underwriting Processes for AI Integration
- Assess Pain Points: Evaluate the underwriting workflow to pinpoint areas that can benefit most from AI, such as risk scoring, data entry, or document processing.
- Set Objectives: Define clear goals, like reducing processing time or improving accuracy, and select specific pilot tasks to test AI impact.
Step 2: Prepare a Data and Compliance Strategy
- Data Collection and Quality Assurance: Gather and clean structured and unstructured data (e.g., medical records, lifestyle data) to ensure a robust dataset for AI.
- Privacy and Security Measures: Use anonymization, encryption, and privacy-preserving AI (e.g., federated learning) to maintain compliance with regulations like HIPAA and GDPR.
Step 3: Select AI Tools and Build a Scalable Tech Infrastructure
- Choose AI Models and Tools: Select appropriate models (e.g., predictive analytics for risk scoring, NLP for document analysis) tailored to underwriting tasks.
- Integrate with Legacy Systems: Ensure seamless compatibility with current underwriting systems to streamline data flow and operations.
Step 4: Pilot AI Solutions and Measure Effectiveness
- Deploy in Pilot Areas: Test AI solutions on a small scale to measure effectiveness, starting with less critical underwriting tasks.
- Establish KPIs: Track metrics like processing time, accuracy, and cost savings to assess AI’s impact, and gather feedback for improvement.
Step 5: Upskill Teams and Foster Cross-Functional Collaboration
- Provide AI Training: Equip underwriters with knowledge of AI tools, focusing on how AI aids decision-making.
- Encourage Collaboration: Facilitate coordination between underwriters, data scientists, and IT teams to optimize AI implementation.
Step 6: Optimize, Scale, and Cultivate AI Adoption
- Monitor and Refine Models: Continuously track performance and refine AI models based on new data and feedback.
- Scale Across Underwriting Processes: Expand AI to additional underwriting tasks after successful pilot outcomes.
- Promote AI Benefits: Foster a culture of innovation by communicating AI’s value in enhancing productivity, accuracy, and customer satisfaction.
Conclusion
AI offers transformative potential to address underwriting challenges in healthcare insurance. By automating data processing, improving risk accuracy, and expediting underwriting workflows, AI enhances efficiency, accuracy, and customer satisfaction in the underwriting process. Healthcare insurers that strategically adopt AI will be well-positioned to offer competitive, tailored insurance products while maintaining regulatory compliance and data security. As the healthcare insurance industry evolves, AI-driven underwriting will play a pivotal role in driving growth and innovation.
Future Outlook
As AI technology advances, the integration of emerging technologies such as explainable AI and advanced data analytics will further enhance underwriting processes, allowing insurers to refine risk models and respond to new challenges in healthcare insurance. Embracing AI will not only streamline underwriting but also set the foundation for a more resilient and customer-focused healthcare insurance industry.