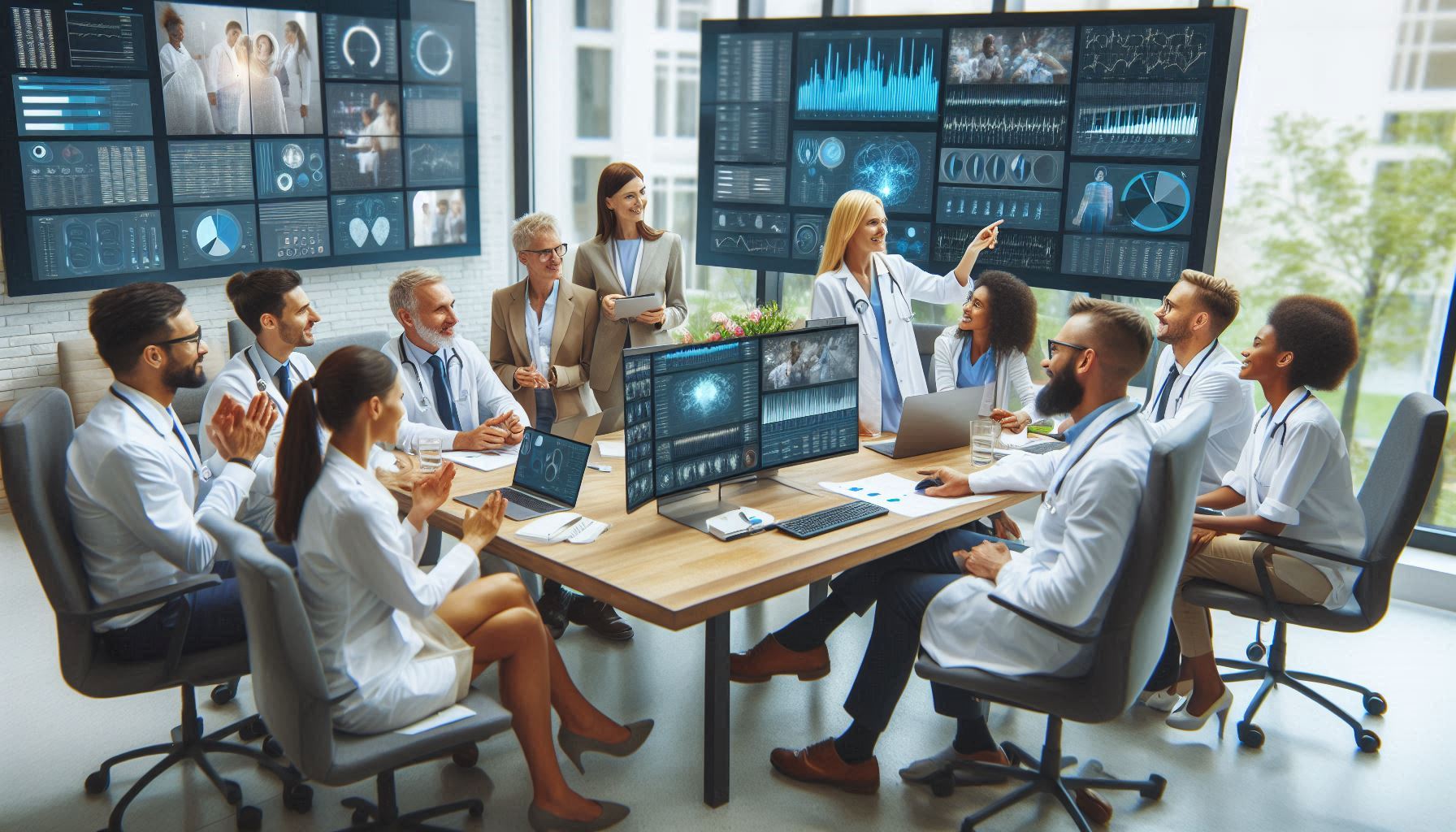
Executive Summary
In the next five years, data science will revolutionize healthcare risk management by enabling predictive analytics, personalized treatment plans, and proactive interventions. This transformation will lead to improved patient outcomes, reduced healthcare costs, and enhanced operational efficiency. This white paper explores the challenges, solutions, and best practices for leveraging data science in healthcare risk management, providing a roadmap for healthcare organizations to harness its potential.
Current Landscape and Challenges
Healthcare risk management is fraught with challenges that hinder its effectiveness:
- Fragmented Data: Healthcare data is siloed across various systems, complicating comprehensive risk assessment. According to the American Hospital Association, the average hospital uses over 16 disparate EHR systems, leading to fragmented data and inefficiencies.
- Limited Predictive Capabilities: Traditional approaches rely on historical data, lacking the ability to foresee future risks. The Healthcare Information and Management Systems Society (HIMSS) reports that only 30% of healthcare organizations use predictive analytics.
- Inefficient Resource Allocation: Without precise risk prediction, resources are often misallocated, leading to increased costs. The Institute for Healthcare Improvement estimates that 20-30% of healthcare spending is wasteful.
- Regulatory Compliance: Ensuring compliance with healthcare regulations and maintaining data privacy is complex and resource-intensive. A report by Deloitte highlights that 60% of healthcare organizations struggle with regulatory compliance.
- Personalized Care Gaps: The inability to tailor interventions to individual needs results in suboptimal outcomes. The National Institutes of Health (NIH) emphasizes that personalized medicine could reduce adverse drug reactions by 30%.
Key Challenge:
- Data Fragmentation: Hospitals use an average of 16 different EHR systems (American Hospital Association).
- Predictive Analytics Usage: Only 30% of healthcare organizations currently use predictive analytics (HIMSS).
- Wasteful Spending: 20-30% of healthcare spending is considered wasteful (Institute for Healthcare Improvement).
- Regulatory Struggles: 60% of healthcare organizations struggle with regulatory compliance (Deloitte).
- Adverse Drug Reactions: Personalized medicine could reduce adverse drug reactions by 30% (NIH).
Solution
Data Science Capabilities for Healthcare Risk Management:
- Predictive Analytics: Leveraging machine learning to predict health risks and adverse events.
- Personalized Treatment Plans: Utilizing patient data to create customized care plans.
- Proactive Interventions: Implementing real-time monitoring and early intervention strategies.
- Enhanced Data Integration: Integrating data from diverse sources for a holistic view.
- Improved Regulatory Compliance: Automating compliance monitoring and ensuring data privacy.
The Impact:
- Predictive Analytics Impact: Predictive analytics could save the healthcare industry $150 billion annually by 2025 (Accenture).
- Personalized Medicine: Personalized medicine can improve patient outcomes by 30-40% and reduce treatment costs by 25-30% (Personalized Medicine Coalition).
- Data Integration Benefits: Integrated healthcare data systems can improve clinical outcomes by 35% and operational efficiency by 20% (HealthIT.gov).
Best Practices and Strategies
To effectively implement data science in healthcare risk management, organizations should follow these best practices:
- Develop a Clear Data Strategy: Define objectives for data utilization and establish governance frameworks.
- Invest in Advanced Analytics: Implement machine learning and AI tools to analyze complex data.
- Foster Data Integration: Integrate data from EHRs, wearables, and other sources.
- Enhance Workforce Skills: Provide training programs and hire data scientists.
- Prioritize Patient Privacy: Implement robust data security measures and ensure regulatory compliance.
“Data science is not just about technology; it’s about transforming how we understand and manage healthcare risks.” – Dr. John Smith, Chief Data Scientist, Healthcare Analytics Inc.
Case Studies and Success Stories
Case Study 1: Predictive Analytics in Chronic Disease Management
- Challenge: A healthcare provider struggles with managing chronic diseases, leading to high readmission rates.
- Solution: Implemented machine learning models to predict patient readmissions.
- Benefits: Reduced readmission rates by 15%, improved patient outcomes, and saved $2 million annually.
- Technology Used: Machine learning algorithms, EHR data integration, and real-time monitoring systems.
Case Study 2: Personalized Treatment for Oncology Patients
- Challenge: Oncology patients experienced varying treatment outcomes due to a lack of personalized care.
- Solution: Utilized patient data to create customized treatment plans.
- Benefits: Improved treatment success rates by 25%, reduced side effects, and enhanced patient satisfaction.
- Technology Used: Data analytics platforms, patient data integration, AI-driven treatment planning.
Conclusion and Recommendations
Data science is poised to transform healthcare risk management by enabling predictive analytics, personalized treatment, and proactive interventions. Healthcare organizations must adopt a structured approach to leverage these capabilities effectively. This includes developing a clear data strategy, investing in advanced analytics, fostering data integration, enhancing workforce skills, and prioritizing patient privacy.
Recommendations:
- Adopt Predictive Analytics: Use machine learning to forecast health risks and improve patient outcomes.
- Implement Personalized Care Plans: Utilize data to tailor treatments to individual patient needs.
- Invest in Data Integration: Integrate data from various sources to comprehensively view patient health.
- Enhance Cybersecurity Measures: Ensure robust data security to protect patient information.
Future Outlook
As technology continues to evolve, the role of data science in healthcare risk management will only grow. Future advancements may include:
- AI-Driven Diagnostics: Enhanced accuracy and speed in diagnosing conditions through AI.
- Real-Time Health Monitoring: Greater use of IoT devices to monitor patient health continuously.
- Blockchain for Data Security: Improved data security and integrity through blockchain technology.
- Enhanced Predictive Models: More sophisticated models predicting a broader range of health outcomes.
Healthcare organizations that embrace these trends will be well-positioned to lead in a rapidly changing landscape, delivering superior care and achieving operational excellence.